2024-05-15 Tunisian Dinar News
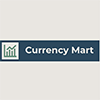
2024-05-14
Summary of Yesterday
- Opening:
- Closing:
- Difference of Opening & Closing:
- Daily High:
- Daily Low:
- Difference of Daily High & Low:
Statistical Measures
- Mean:
- Standard Deviation:
Trend
Overall Trend in Exchange Rates
The overall trend of the exchange rate for TND (Tunisian Dinar) over the provided time period appears to be relatively stable with slight fluctuations. The initial rate started from 0.43621 and ended at 0.43647 in the final observation. There seem to be some minor increases and decreases in between these data points. However, it is essential to note that without further statistical tests or visual representation, the argument for trend stability remains preliminary.
Seasonality or Recurring Patterns
Seasonality or recurring patterns in time-series data usually refer to predictable and repeating patterns over the given time period. In this context, the exact identification of any seasonality or recurring pattern could be more challenging due to the minute intervals of the time stamps and the fractional unit of the exchange rate, which alone might not evoke visible seasonality. However, when checking the major time intervals such as at the top of the hour, some slight fluctuations could be observed, but these do not seem to present a clear pattern of regular and predictable change. In-depth time-series analyses such as spectral analysis or autocorrelation analysis can be treated as potential next steps in identifying these patterns.
Outliers Analysis
Determining outliers in such kind of high-frequency financial time-series data can be very subject to the adopted definition of the outlier. A simplistic approach, like defining any rate that deviates from the median by more than a certain number of standard deviations, might suggest certain points as possible outliers. However, it is important to mention that a more robust statistical algorithm which considers the complex nature of time series data (e.g., ARIMA modeling) would be more suitable for adequately detecting outliers. Please note that without implementing these tests, it is not possible to comprehensively identify the presence of outliers in your provided dataset.
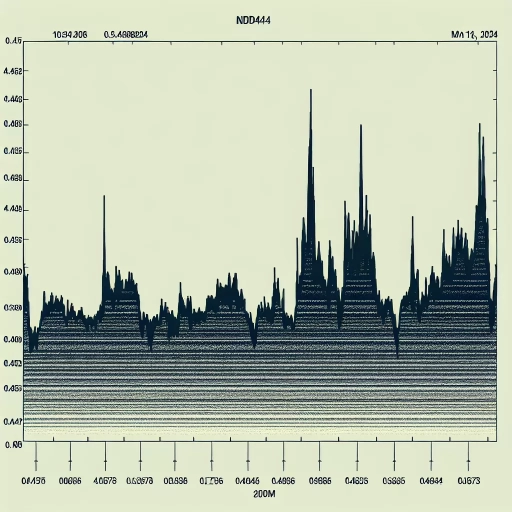