2024-04-22 Sudanese Pound News
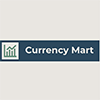
2024-04-21
Summary of Last Week
- Opening:
- Closing:
- Difference of Opening & Closing:
- Daily High:
- Daily Low:
- Difference of Daily High & Low:
Statistical Measures
- Mean:
- Standard Deviation:
Trend
Overview of the dataset
This is a time-series dataset spanning from March 22, 2024, to April 19, 2024. The data points mostly appear in recurrent intervals, with several instances where multiple data points occur at the same timestamp. The response variable, labeled 'SDG,' represents the exchange rate at each timestamp. As described, the timestamps generally fall at hourly intervals, although there are some instances of larger gaps where data for several hours is missing.
Trends in the dataset
The overall trend of the dataset shows fluctuations in the value of 'SDG' across the designated period. These fluctuations point towards periodical rise and fall in the rates, but overall, there doesn't seem to be a strong upward or downward trajectory during this time frame. For most of the dataset, the 'SDG' values range between 0.00225 and 0.00235, with average around 0.00231.
Seasonality
While this dataset does not provide multiple years of data, which would be ideal for determining seasonality, some recurring patterns can be noted. For instance, there are several cases of the exchange rate remaining consistent or nearly so for a few timestamps before changing again. This could be potentially due to trading sessions but specific seasonal trends cannot be positively identified without further analysis and more data.
Outliers
The data does not show extreme fluctuations or data points that stand far apart from others. Additionally, the relatively narrow range of the exchange rate values suggests that there do not appear to be significant outliers in the data. Any moderate fluctuations could be attributed to normal market volatility rather than unusual or unexpected changes.
While this analysis gives you a basic understanding of the trends, patterns, and potential outliers in your dataset, a more in-depth analysis may require more advanced statistical techniques or even machine learning algorithms. This can also assist in confirming any identified patterns and detecting others that may not be immediately apparent.
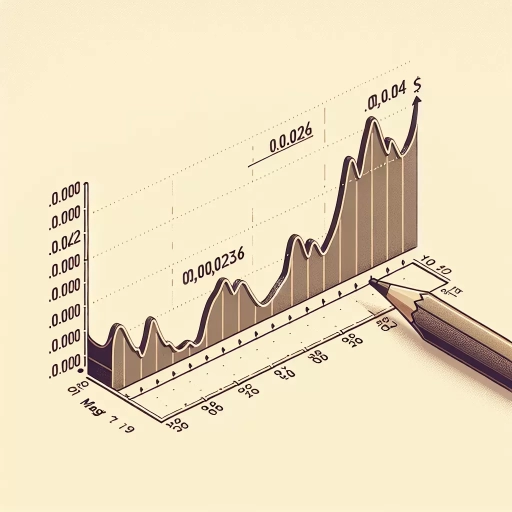