2024-05-03 Singapore Dollar News
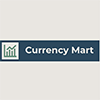
2024-05-02
Summary of Yesterday
- Opening:
- Closing:
- Difference of Opening & Closing:
- Daily High:
- Daily Low:
- Difference of Daily High & Low:
Statistical Measures
- Mean:
- Standard Deviation:
Trend
Overall Trend Analysis
From the provided dataset, it appears that the SGD exchange rate witnessed minor fluctuations throughout the entire timespan. There's no clear ascending or descending trend recognizable, and it suggests the rate remained relatively stable. This could be due to a variety of factors like balanced demand and supply, unchanging monetary policies, among others.
Seasonality and Recurring Patterns
As for recurring patterns and seasonality, a comprehensive assessment of the time series data doesn't suggest any prominent cyclical changes within the daily observation. Additional information such as hourly, weekly, or monthly data might show a more detailed view of the possible cyclical properties or inflection points. However, based on the data provided, many of the changes seem to be responsive to short-term factors or random fluctuations rather than regular, season-specific impacts.
Outliers
The data given here shows a few sharp fluctuations, but it's hard to identify outliers without more context or statistical analysis to anchor a definition of what constitutes an 'outlier' in this setting. It's notable that there are instances where the exchange rate has experienced small spikes and decreases; however, these fluctuations fit within the general ebb and flow of the data and therefore do not meet the typical criteria of an outlier.
Additional Considerations
Even though you've requested not to consider such, the forex market is intrinsically influenced by external factors like market opening/closing hours, weekends/holidays, or the release of key financial news and reports. It's vital to treat financial time series analysis with a grain of salt as a result of its high susceptibility to many known and unknown variables.
Lastly, given the high-frequency nature of fx market data, more advanced time series analysis methods like GARCH or ARIMA and machine learning techniques may help uncover hidden structures or predictive power within the series. Although such deeper analysis is outside the scope of the current request.
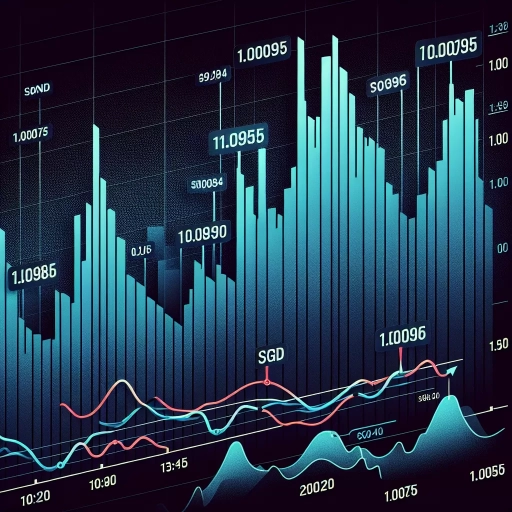