2024-04-23 Singapore Dollar News
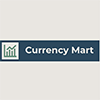
2024-04-22
Summary of Last Month
- Opening:
- Closing:
- Difference of Opening & Closing:
- Daily High:
- Daily Low:
- Difference of Daily High & Low:
Statistical Measures
- Mean:
- Standard Deviation:
Trend
Analysis of provided Time-Series Data
Before jumping into the analysis of the dataset, let's clarify the structure of the given data. The dataset is given in time-series format (YYYY-MM-dd hh:mm:ss), where timestamps likely represent every 5 minutes; following each timestamp, the corresponding Singapore Dollar (SGD) exchange rate is provided. The main goal is to analyze trends, identify seasonality, and locate any outliers in the data.
Overall Trend Analysis
From an initial glance, the currency exchange rate appears to be fluctuating within a specific narrow range. The start and end values do not differ significantly, suggesting a relatively stable period with no dramatic increase or decrease trend. It started from 1.0088, and the last value recorded is 1.00625 which is slightly decreased, but the scale of change is small. However, to fully understand the overall trend, additional data processing and a more in-depth review would be necessary, probably involving the use of trend lines and moving averages.
Seasonality and Recurring Patterns
Detecting seasonality or recurring patterns is slightly more challenging due to the apparent random nature of the fluctuations viewed at the minute scale this dataset provides. The fluctuations don't seem to show repetitive or obvious cyclical patterns, suggesting the need for a more rigorous method, like autocorrelation or Fourier analysis approach, to accurately identify any potential periodic patterns or seasonality.
Identification of Outliers
At the glance, the dataset does not indicate a presence of significant outliers—values that are dramatically lower or higher than the typical exchange rates. However, as with the above analyses, this would need to be confirmed by more rigorous statistical analysis. Usually, methods like standard deviations, boxplots, or IQR (Interquartile Range) can be used to detect outliers effectively and are recommended for confirmation, especially in financial time-series data analysis, which can be heavily impacted by outliers.
Please be reminded that this analysis is based on a quick visual assessment of the dataset. For a more rigorous and precise understanding of the data, advanced statistical methods and machine learning algorithms should be performed.
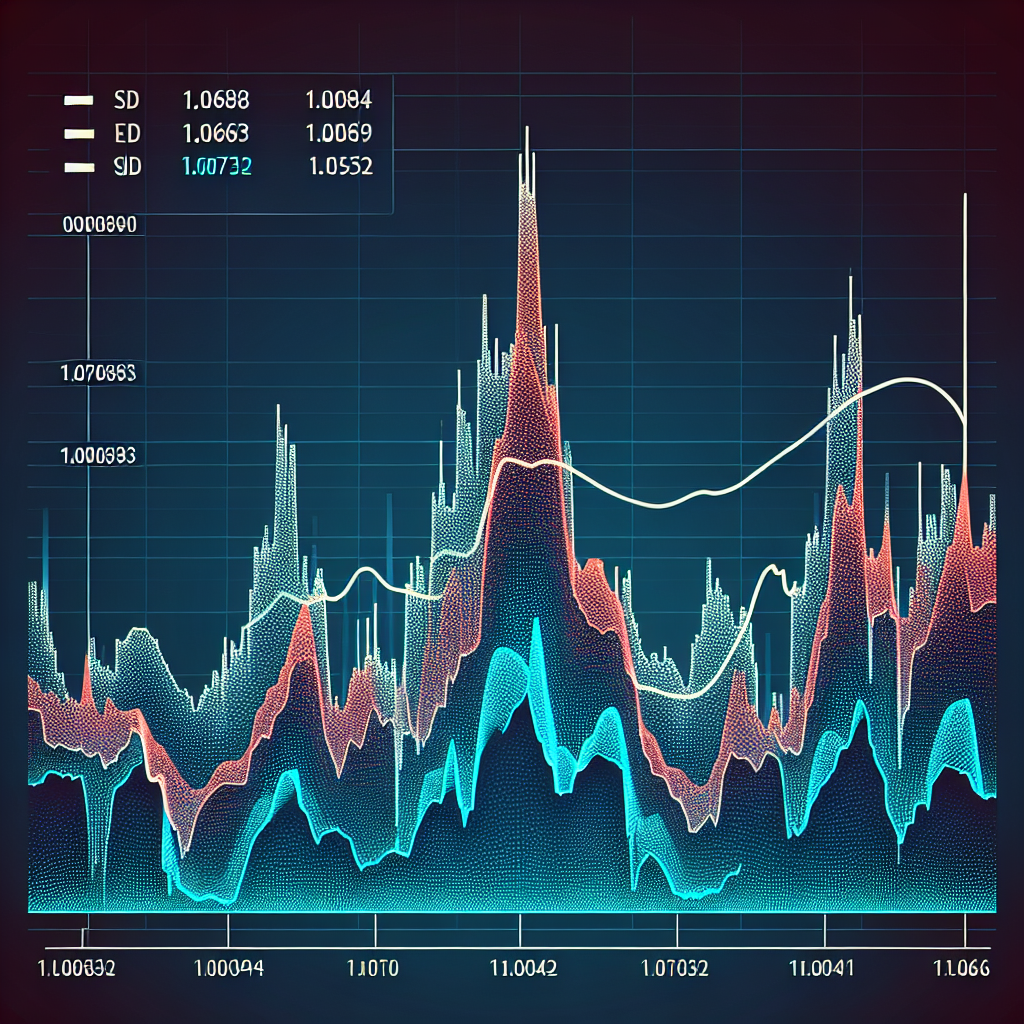