2024-05-13 Nuevo Sol News
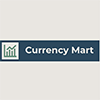
2024-05-12
Summary of Last Week
- Opening:
- Closing:
- Difference of Opening & Closing:
- Daily High:
- Daily Low:
- Difference of Daily High & Low:
Statistical Measures
- Mean:
- Standard Deviation:
Trend
1. Understanding the overall trend of the exchange rates
From the dataset provided, the overall trend of the exchange rate can be seen. The exchange rate started at 0.36892 on 2024-04-12 02:00:02 and finished at 0.37036 on 2024-05-10 12:00:02. This indicates a slight increase in the exchange rate over the specified period. The range of the exchange rate during this time was from approximately 0.363 to 0.373, highlighting the volatility within the market. Aside from regular fluctuations, there is no clear continuous uptrend or downtrend visible from the data.
2. Identifying any seasonality or recurring patterns in the changes of exchange rates
In identifying any seasonality or recurring patterns, a more detailed and complex analysis would be needed typically involving tools such as autocorrelation plots, spectral density analysis, or Fourier transforms. However, based on simple observation from the given data, there appear to be no blatant periodic patterns or seasonality. Keep in mind that exchange rates can be influenced by a variety of unpredictable and uncontrollable macroeconomic factors, making it inherently hard to pinpoint definitive seasonal patterns.
3. Noting any Outliers
Looking at the data a few points stand out as potential outliers. These are instances where the exchange rate differs significantly from what would be expected based on the trend. In particular, the spike in rates to about 0.373 on 2024-04-12, and the dip to around 0.363 on 2024-04-26. These outliers could be an effect of several factors not included in the given data, such as key political events, changes in tariff laws, or drastic shifts in supply and demand. In financial analysis, identifying these outliers can provide invaluable insight into understanding how susceptible the market is to sudden changes.
Note that a thorough outlier detection might require more advanced statistical techniques beyond visual inspection, including Z-scores, the IQR method, or anomaly detection algorithms.
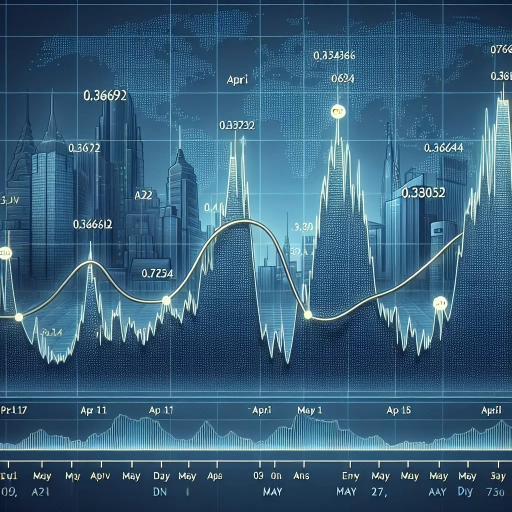