2024-05-13 Nepalese Rupee News
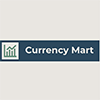
2024-05-12
Summary of Last Week
- Opening:
- Closing:
- Difference of Opening & Closing:
- Daily High:
- Daily Low:
- Difference of Daily High & Low:
Statistical Measures
- Mean:
- Standard Deviation:
Trend
1. Understanding the overall trend of the exchange rates
The dataset provided essentially follows a similar pattern of fluctuation across the period under review. The exchange rates do not show a major ascending or descending trend; instead, they maintain stability with minor variations. The NPR exchange rate began at a value of 0.01028 on the 12th April 2024 and concluded at a value of 0.01023 on the 10th May 2024. The dataset registered slight increase and decrease in rates throughout, but the overall trend indicates a stable exchanging rate over the period shown under analysis.
2. Identifying any seasonality or recurring patterns in the changes of exchange rates
Upon investigation of the data, it seems that there is no clear seasonality or recurring patterns observed in the change of exchange rates. The rates across the period do not display consistent peaks or troughs, which signifies the absence of seasonality. Moreover, the rates do not demonstrate any evidence of consistent cycles or patterns recurring at regular intervals. Hence, it can be concluded that the data lacks noticeable seasonality or recurring patterns in the changes of exchange rates.
3. Noting any outliers, or instances where the exchange rate differs significantly from what would be expected based on the trend or seasonality
In the given dataset, it's hard to identify outliers as the exchange rate remains relatively stable, with very minor fluctuations. These minute fluctuations are typical in financial time-series data like exchange rates. Moreover, since there aren't any significant operational shifts or drastic changes observed in the dataset, it's safe to conclude that there are no outliers that differ markedly from the general trend or seasonality.
Please note that this analysis is based on data pattern observations. A more detailed investigation can lead to different insights and may require the application of sophisticated time-series analysis methodologies or machine learning models.
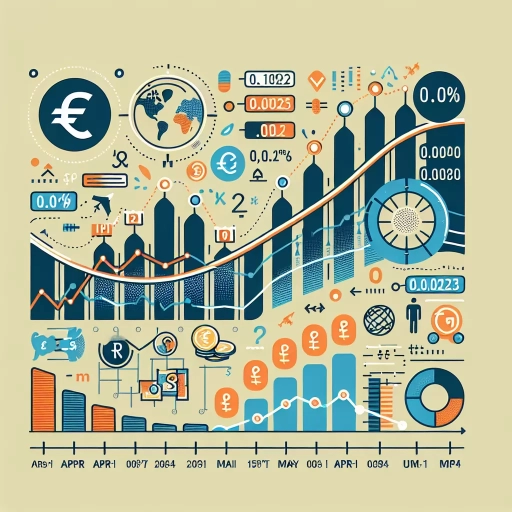