2024-04-29 Latvian Lats News
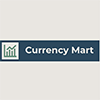
2024-04-28
Summary of Last Week
- Opening:
- Closing:
- Difference of Opening & Closing:
- Daily High:
- Daily Low:
- Difference of Daily High & Low:
Statistical Measures
- Mean:
- Standard Deviation:
Trend
Analysis of Exchange Rates Over Time
The exchange rates data provided contains values ranging from a minimum of 2.22909 to a maximum of 2.28623. The data spans multiple dates in 2024, from March 29th to April 26th. Upon visual inspection, the exchange rates exhibit some variability but do not seem to follow a clear increasing or decreasing trend over the entire period.
Overall Trend
At the beginning of the period (March 29th), the exchange rates started around 2.24, reached a minimum around April 4th (at 2.22909), rebounded and fluctuated, and peaked on April 16th at 2.28623. Thereafter, they generally decrease with some fluctuations and end at 2.25756 on April 26th. There seems to be no clear upward or downward trend over the entire period.
Seasonality and Recurring Patterns
Considering the recurrent patterns, this analysis doesn't reveal any clear daily or weekly seasonality. That is, it's not obvious to state that there are consistent patterns where the exchange rate predictably rises or falls at certain times of the day or certain days of the week. There are periods of relative stability, followed by periods of higher volatility where the rate increases or decreases more abruptly.
Outliers
There wasn't any clear presence of extreme outliers where the exchange rate differed significantly from the pattern. However, there are periods of sharp increases and decreases that could be considered as unusual. For instance, the sharp increase on April 16th, reaching the peak value of 2.28623, and the subsequent decline could be considered as unusual movements.
In summary, while the exchange rate data does exhibit variability over time, it doesn't show obvious signs of a consistent trend, clear seasonality, or significant outliers. It shows a complex pattern influenced by a variety of possible factors. Given this complexity, advanced modeling techniques like time series analysis or machine learning may be required to understand and predict future movement more accurately.
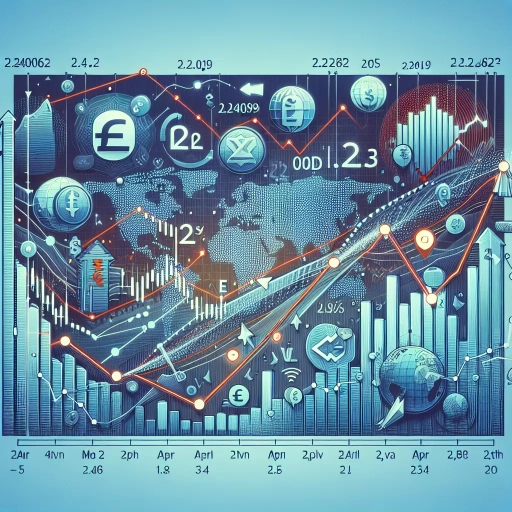