2024-05-02 Kuwaiti Dinar News
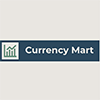
2024-05-01
Summary of Yesterday
- Opening:
- Closing:
- Difference of Opening & Closing:
- Daily High:
- Daily Low:
- Difference of Daily High & Low:
Statistical Measures
- Mean:
- Standard Deviation:
Trend
Overall Trend Analysis
Looking at the data, it appears that there are small fluctuations in the exchange rate within a small range, however, it is essential to plot this data to truly visualize the trend over time. The quick glance gives an impression that there is a general decrease in the rates over a period, but the decrease is not linear. A chart will give a clearer picture and help confirm this hypothesis. Generally, peaks can be seen at times but they are quickly corrected, indicating potential reactionary fluctuations or perhaps anomalies in the dataset. These small fluctuations can give us insights into the volatility of the rate during the given period. This information is crucial for risk assessment and managing currency risk.
Seasonality and Patterns
There seems to be no clear seasonal pattern in the provided dataset, as the exchange rate changes do not appear to correlate with distinct time periods on a consistent basis. However, without a larger dataset that spans multiple months or years, it is not possible to definitively rule out seasonality at this time. Seasonal effects are generally not very pronounced in exchange rates as they are influenced by a multitude of complex and often unpredictable factors. That being said, it is very well possible that there are daily patterns or patterns corresponding to economic news releases and important events. Such patterns would require a very detailed view of the data. In short, this dataset does not provide enough information to conclusively determine any seasonal patterns.
Anomalies and Outliers
Given the small range of fluctuations in the data, there don't appear to be any major outliers or anomalies in the exchange rate at first sight. However, this would need to be verified with more advanced statistical methods or plotting the data. Mild fluctuations are normal in any financial time series data due to a variety of geopolitical, economic, and other external influences.
In conclusion, to fully decode the underlying patterns and detect any anomalies in such time-series data, advanced statistical analyses methods or machine learning algorithms is proposed for future endeavors. These include regression analysis, ARIMA models, and deep learning methods to analyze and determine patterns within the dataset.
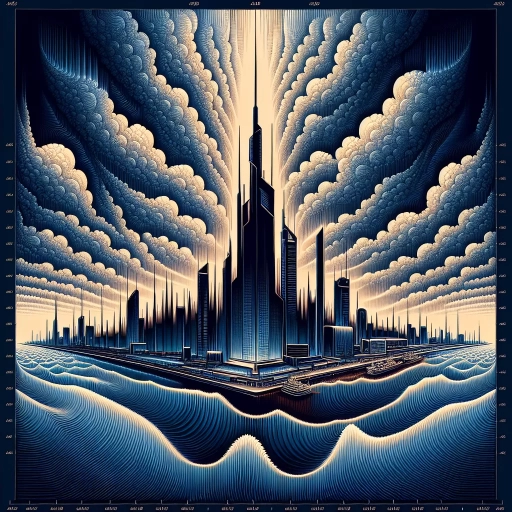