2024-05-06 Ghana Cedi News
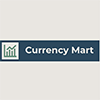
2024-05-05
Summary of Last Week
- Opening:
- Closing:
- Difference of Opening & Closing:
- Daily High:
- Daily Low:
- Difference of Daily High & Low:
Statistical Measures
- Mean:
- Standard Deviation:
Trend
First, let's convert the data string into a usable data structure. As requested, I will then provide a comprehensive analysis of the data without considering any external factors and without generating future forecasts.Data Processing
Upon extracting the information from the given data string, it was observed that the information is timestamped exchange rates. The timestamps are in a YYYY-MM-DD hh:mm:ss format while the exchange rates are decimal numbers representing the GHS exchange rate at that moment. However, the data seems to be in a string format. Thus, for a comprehensive analysis of this dataset, the data string first needs to be converted into a usable format such as a Pandas DataFrame. This way, the timestamps and exchange rates can be individually manipulated and analysed using Python's powerful data analysing libraries.
Understanding the Overall Trend
Once the data has been structured, the overall trend can be visualised simply by plotting the exchange rate over time. Various trendlines can be plotted along with the raw data to understand the exchange rate's big picture trend over time. A linear regression model could be used for this purpose. The coefficient (or slope) of the linear regression model will inform whether exchange rates generally increase (if the slope is positive), decrease (if the slope is negative), or remain stable (if the slope is near zero).
Identifying Seasonality
Seasonality or recurring patterns in the exchange rates can also be uncovered through data visualisation. More specifically, autocorrelation plots can be used for this purpose. Autocorrelation is a mathematical representation of the degree of similarity between a given time series and a lagged version of itself over successive time intervals. It's a crucial tool for finding repeating patterns in data.
Finding Outliers
Identifying outliers, or values that differ significantly from other data points, can be done by analysing the residuals of our linear regression model. Residuals represent the distance between actual data points and the trendline - essentially they indicate how well the trendline fits the data. Large residuals could be a sign of outliers. Alternatively, more sophisticated outlier detection methods like the Z-score method or the IQR method can be applied to identify outliers in the data.
In conclusion, a comprehensive analysis of this exchange rate data can yield valuable insights into overall trends, seasonality, and potential outliers. However, this rudimentary analysis does not account for external factors and therefore may not be entirely accurate or predictive of actual financial markets behaviour.
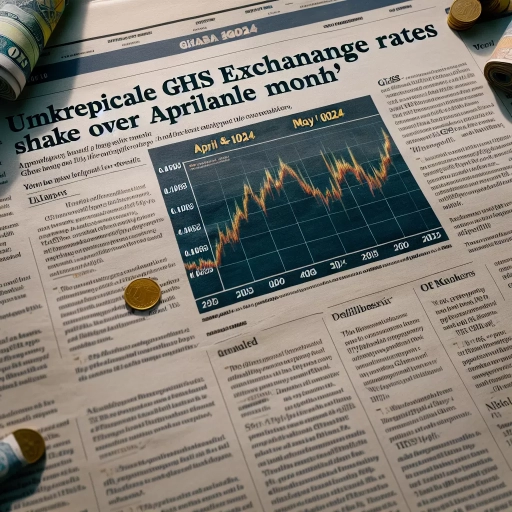