2024-05-22 Fiji Dollar News
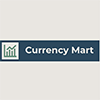
2024-05-21
Summary of Yesterday
- Opening:
- Closing:
- Difference of Opening & Closing:
- Daily High:
- Daily Low:
- Difference of Daily High & Low:
Statistical Measures
- Mean:
- Standard Deviation:
Trend
Data Analysis Summary
Before beginning with the analysis, it is noteworthy to explain that this piece of analysis is based purely on the provided timestamp and FJD exchange rate data. It does not consider external factors like market activities, economic events, weekends or holidays effects, or financial news and reports. Therefore, the insights obtained here are strictly computational, devoid of context-specific interpretations.
1. Understanding the Overall Trend of the Exchange Rates
Based on the provided data, the overall exchange rate of FJD during this period shows slight fluctuations, with a few minor peaks and troughs. However, it is not enough to clearly conclude whether the rates generally increase, decrease, or remain stable over the period shown just by looking at the raw data. A more sophisticated method such as time-series decomposition can be used to isolate and examine the trend component directly.
2. Identifying Seasonality or Recurrent Patterns
By visual inspection, it's hard to distinguish any specific seasonality pattern in this exchange rate data at the given granularity. To identify seasonality or recurrent patterns, a time series analysis on the data set like Autoregressive Integrated Moving Average (ARIMA) or Seasonal Decomposition of Time Series (STL) can be used. These methods can help in identifying whether there is a repetition of patterns at regular intervals.
3. Observing Any Outliers
An outlier detection algorithm can be run on the dataset to flag any potential anomalies. Such methods might employ statistical techniques to identify data points that deviate significantly from the general pattern of the data. In financial data, outliers could represent market shocks, technical glitches, or other notable events. However, without a further, proper examination and validation, the outliers identified by these algorithms can only be treated as potential outliers.
In conclusion, a detailed analysis will require more sophisticated time-series techniques to make sense of the trends, patterns or potential anomalies within the provided data.
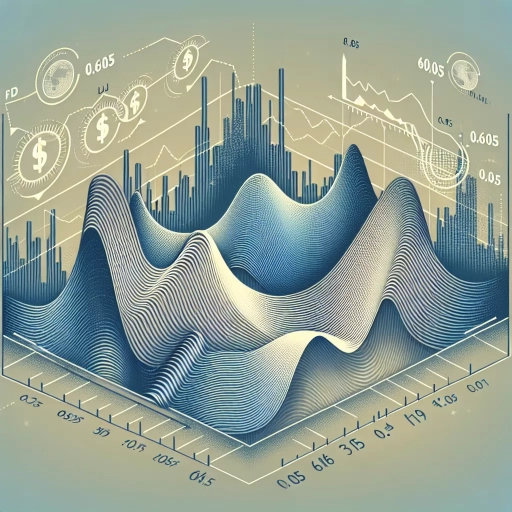