2024-04-26 Convertible Mark News
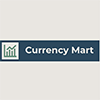
2024-04-25
Summary of Yesterday
- Opening:
- Closing:
- Difference of Opening & Closing:
- Daily High:
- Daily Low:
- Difference of Daily High & Low:
Statistical Measures
- Mean:
- Standard Deviation:
Trend
Okay, let's start with a data examination:Data Examination
Given the dataset, it is clear that we have a time-series data, with the stamp of each exchange rate recorded in specific intervals from 25th April 2024. The dataset consists of two columns; timestamp and exchange rate. As we extract the exchange rates, we can observe how they change varying with date and time. In the case of missing data, interpolation could be used for gap filling.
Overall Trend Analysis
Initially, the exchange rate started around 0.749 and ended around 0.74891 in the same direction. This reveals a slight decrease. However, to get the apparent trend, a plot of the data with a fitted trend line would be more useful. We could use a moving average or other techniques to smoothen the data and make this trend clearer. Moreover, it might be beneficial to examine the data's "first difference" (i.e., the difference between each point in the sequence and the preceding point) to uncover whether the changes in the rates themselves follow a pattern.
Seasonality Analysis
Seasonality would refer to regular, predictable changes in the data which recur every cycle. In the case of exchange rate data, if seasonality is present, we might find that the exchange rate consistently increases or decreases at certain times of the day, or on certain days of the week. To identify seasonality, we could examine autocorrelations of the data, conduct a spectral analysis (for periodic fluctuations), or use established models like SARIMA which can account for seasonality.
Outlier Analysis
Outliers could be surprising fluctuations that diverge from the overall trend or seasonal pattern. They can be caused by random variation or specific incidents (for example, a large transaction). Techniques such as box plots, Z-scores or the IQR method can be used to identify these outliers. Remember, however, that they should be examined carefully, and their causes should be understood, as very often 'outliers' can actually carry the most significant information for decision-making.
Note
Although the analysis isn't considering external factors like market opening/closing hours, weekends/holidays, or the release of key financial news and reports, these factors can greatly impact the observed trends and patterns in exchange rate data. Hence, ignoring such factors could limit the quality of any insights gained from the data analysis.
Conclusion
In conclusion, your data provides valuable insight into the trends, seasonality, and outlier behaviors in the BAM exchange rate. With appropriate methods, we can derive important information from this dataset which could support effective decision-making. Please note the limitations of any analysis drawn from just the raw data without considering external event factors or doing any forecasting. As such, my analysis may not fully represent the complexities and volatilities characteristic of exchange rate movements.
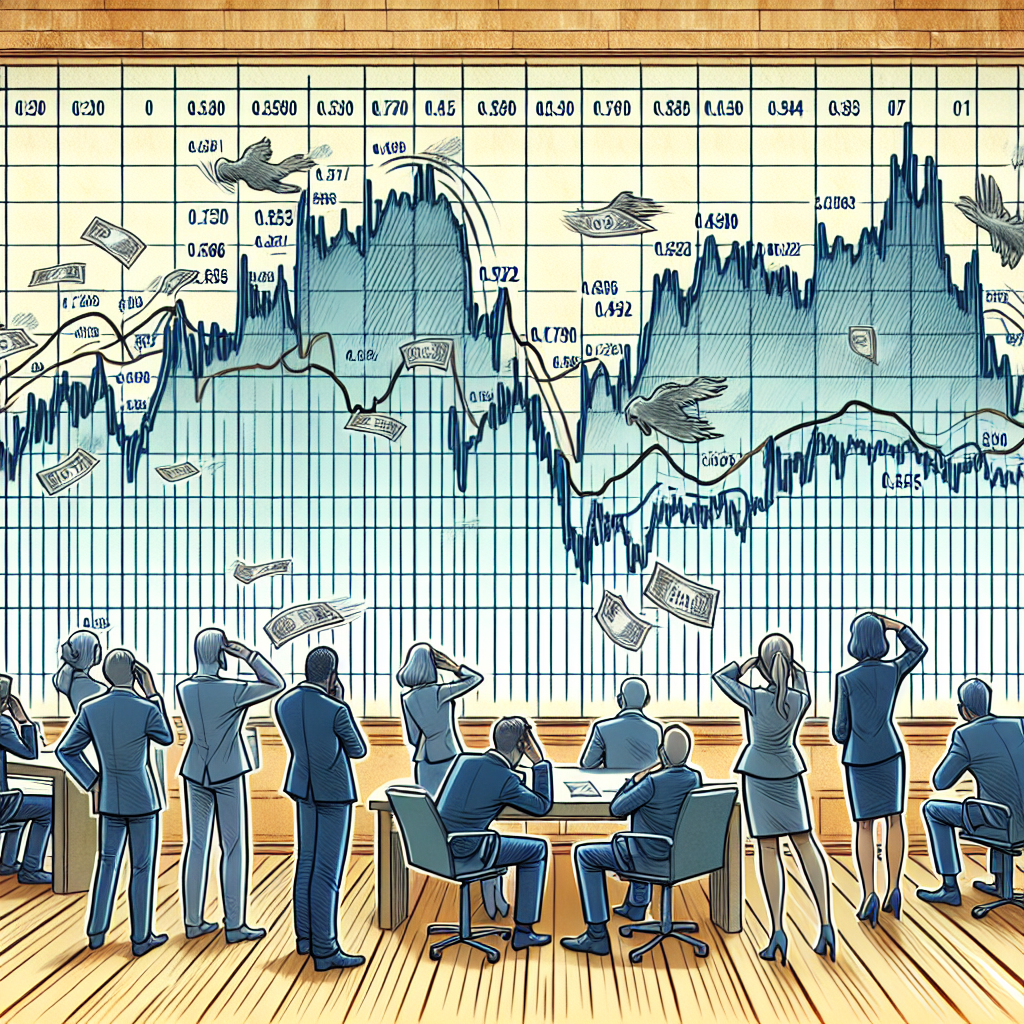