2024-05-13 Brazilian Real News
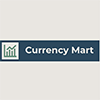
2024-05-12
Summary of Last Week
- Opening:
- Closing:
- Difference of Opening & Closing:
- Daily High:
- Daily Low:
- Difference of Daily High & Low:
Statistical Measures
- Mean:
- Standard Deviation:
Trend
To carry out a comprehensive analysis of the provided dataset, we need to first organize the data correctly. Currently, timestamps of data points and associated exchange rates are provided in a flat format. Let's structure these timestamps and exchange rates into two distinct columns for a clearer understanding before diving into intricate analysis: Date: Refer to the exact timestamp of a given record. BRL: Refer to the Brazilian Real’s exchange rate at the corresponding timestamp. After structuring the dataset appropriately, let's perform the analysis focussing on the three main objectives:1. Understanding the Overall Trend of the Exchange Rates
To understand the overall trend of the exchange rate over the time period provided, we need to visualize data through a time series line plot, where the x-axis will represent the timeline and the y-axis will represent the BRL exchange rates. Observing the upward or downward movement of the line will help analyze whether the rates are generally increasing, decreasing, or remaining stable over the time period. In essence, the line's slope determines the trend; a positive slope represents an increasing trend, a negative slope represents a decreasing trend, and a near-flat line represents a stable trend.
2. Identifying Seasonality or Recurring Patterns
To identify any seasonality or recurring patterns in exchange rates, we can utilize techniques such as autocorrelation and Fourier transforms. Autocorrelation involves the correlation of the time series with a lagged version of itself. Large spikes at certain lags could help identify seasonality. A Fourier transform, on the other hand, could help highlight patterns at certain frequencies. Peaks at certain frequencies would indicate repeating patterns over those time intervals. We could also attempt to observe seasonality by visual inspection of the time series plot. However, this might not always be accurate for longer, more complex time series.
3. Noting Outliers in the Data
Outliers can significantly impact our understanding and modelling of the data. We need to identify these so that they can be handled appropriately. Outliers can be detected by several methods in time series analysis such as using Z-Score, Modified Z-Score, IQR method, and the Hampel Filter. These methods calculate the deviation from central tendency, variation, and rareness of a data point which can then classify a point as an outlier. While handling outliers, one can either remove outliers or fill them with statistical measures like mean, median, or mode.
It's essential to note that results might differ when additional dimensions such as external factors like market opening/closing hours, weekends/holidays, and release of key financial news and reports are considered. The approach shared above is strictly a time series analysis based on the dataset provided without any assumptions or consideration of external events and factors. It's also important to note that this analysis does not generate any forecast for future rates.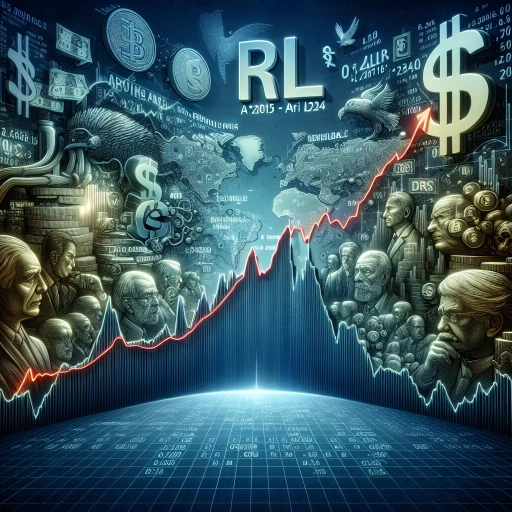