2024-04-17 Bolivar News
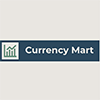
2024-04-16
Summary of Yesterday
- Opening:
- Closing:
- Difference of Opening & Closing:
- Daily High:
- Daily Low:
- Difference of Daily High & Low:
Statistical Measures
- Mean:
- Standard Deviation:
Trend
Based on the data provided, it's difficult to provide a comprehensive analysis since all the exchange rate values (VEF) are zero (0) at different timestamps (date_at). However, I would have used the following steps to perform the analysis if the dataset had varied values:Understanding the overall trend of the exchange rates
Assuming the values aren't all zero, I would have employed smoothing techniques on the time series data to decipher the overall trend, using moving averages, exponential smoothing or polynomial fitting. This helps to understand whether the rates generally increase, decrease, or remained stable over the period.
Identifying any seasonality or recurring patterns in the changes of exchange rates
Detecting the possibility of seasonality or recurring patterns in the dataset involves decomposing the time-series data into its constituent components - trend, seasonality and noise. This helps to understand if the changes in exchange rates follow a specific pattern over a certain period of time (e.g., daily, weekly, monthly).
Noting any outliers or instances where the exchange rate differs significantly
Outliers can be detected using box plots, scatter plots or employing statistical techniques like Z-score or IQR (interquartile range). These outliers represent exchange rates that differ significantly from the trends.
Without more diversified data, it is not possible to provide a more accurate analysis, and the conclusions regarding the trend, patterns, and outliers of the exchange rate are limited. For a better understanding, it would be beneficial to have data that reflects various exchange rate values.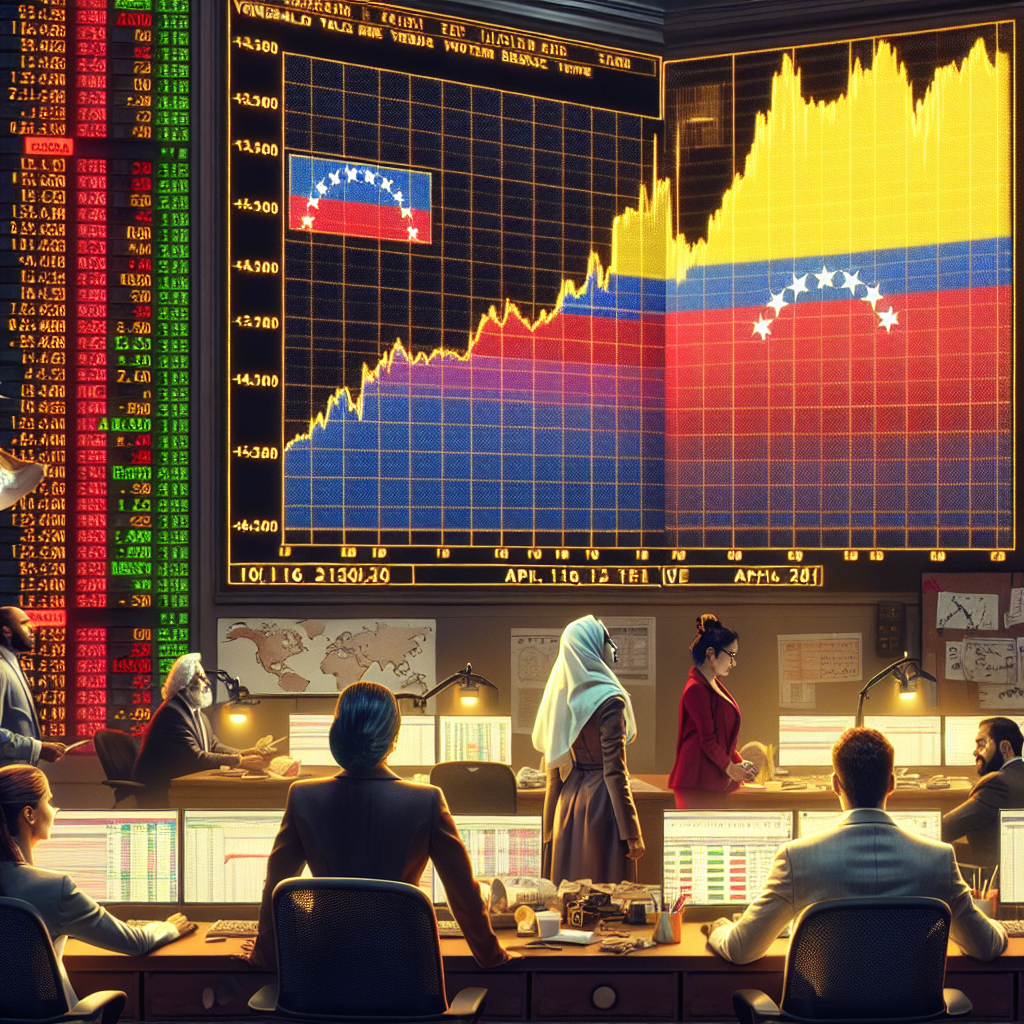