2024-05-08 Azerbaijanian Manat News
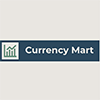
2024-05-07
Summary of Yesterday
- Opening:
- Closing:
- Difference of Opening & Closing:
- Daily High:
- Daily Low:
- Difference of Daily High & Low:
Statistical Measures
- Mean:
- Standard Deviation:
Trend
1. Understanding the Overall Trend
Upon studying the provided dataset, it appears that the exchange rate has experienced both highs and lows throughout the time period. However, it is hard to confirm a consistent increasing or decreasing trend over the time period as fluctuations are noticeable. It is essential to plot the data points over time to better visualize any overarching trend or direction. The calculation of moving averages might also provide a clearer picture of the overall trend, by smoothing out short-term fluctuations and highlighting longer-term trends or cycles.
2. Seasonality or Recurring Patterns
Clear seasonality or recurring patterns in the dataset are difficult to identify without thorough data visualization and time-series decomposition analysis. We could use advanced time-series analysis techniques, such as Autoregressive Integrated Moving Average (ARIMA) models, to help in identifying recurring patterns in our data. By breaking down the time series data into trend, seasonal, and residual components, we may observe if there is any recurring pattern in a particular interval.
3. Outliers
Identifying exact outliers within this dataset would require a more in-depth statistical analysis, involving setting an acceptable range beyond which values would be deemed outliers. This could be calculated using methodologies like the Interquartile Range (IQR) or Z-score. Nevertheless, without applying these techniques, outlier identification remains challenging. Still, we can observe some instances where significant jumps in the exchange rate occur; these could potentially be outliers but would need statistical confirmation.
Note: This analysis is purely based on data exploration and initial observations. For a more comprehensive understanding and actionable insights, advanced time-series analysis and statistical modeling would be recommended. Factors such as market opening/closing hours, weekends/holidays, and the release of key financial reports have not been considered here as per the requirements but should be taken into account for a real-world, comprehensive financial analysis.
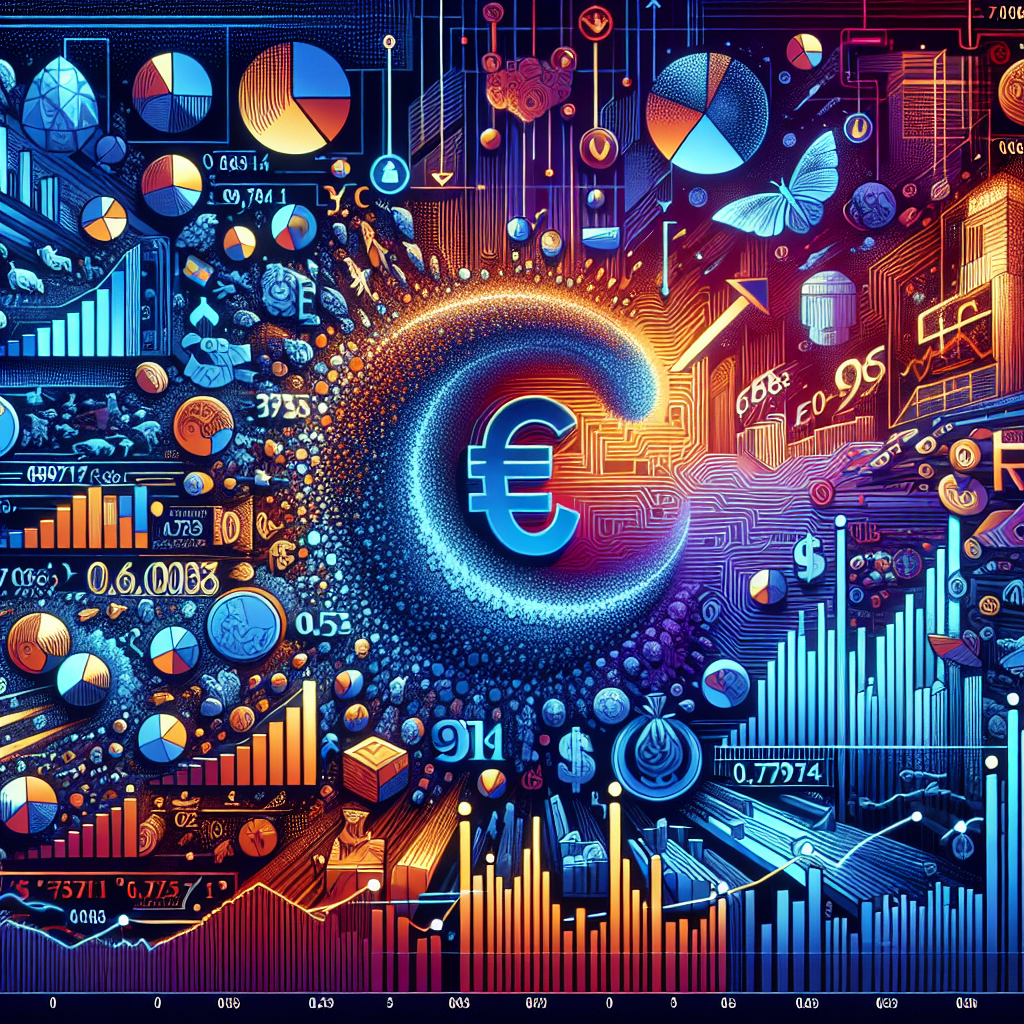