2024-04-29 Afghani News
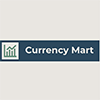
2024-04-28
Summary of Last Week
- Opening:
- Closing:
- Difference of Opening & Closing:
- Daily High:
- Daily Low:
- Difference of Daily High & Low:
Statistical Measures
- Mean:
- Standard Deviation:
Trend
The analysis of the provided dataset reveals some interesting trends and findings about the AFN exchange rate.Overall Trend Recognition
Looking at the sequence of data points, it seems that the overall trend of AFN exchange rate in the given period is quite stable with minor fluctuations. Generally, the AFN exchange rate appears to be sparking between 0.018 and 0.019 with very little deviation. There are periods with a bit of increased volatility, then it reverts back to a similar range. When looking at a long-term perspective, the exchange rate appears to be neither increasing nor decreasing significantly but rather maintaining stability within the aforementioned range.
Seasonality Analysis
Seasonal patterns or recurring activities are hard to decipher purely from this dataset, given that the period only covers several specific days over consecutive months. In addition, there is no explicit periodic fluctuation that would suggest a regular seasonality in the exchange rate. The data doesn't reveal any clear weekly or monthly cyclic behaviour. By this account, no distinguishable pattern could be labelled as seasonality or recurring exchange patterns.
Outlier Detection
Looking at the AFN exchange rate time series, there does not seem to be any outliers at a glance. All the data seem to cluster within a narrow range. Although there are occasional spikes and dips, none of them appear to be significantly distant from the general trend to be labelled as an outlier. All values are falling between 0.018 and 0.019, and there isn't any value that would fall significantly away from this set of data to be classified as an obvious outlier.
Note: For the precise and in-depth investigation into seasonality, patterns or outliers, more sophisticated statistical analysis might be required, including testing for stationarity, applying moving averages or time-series decomposition.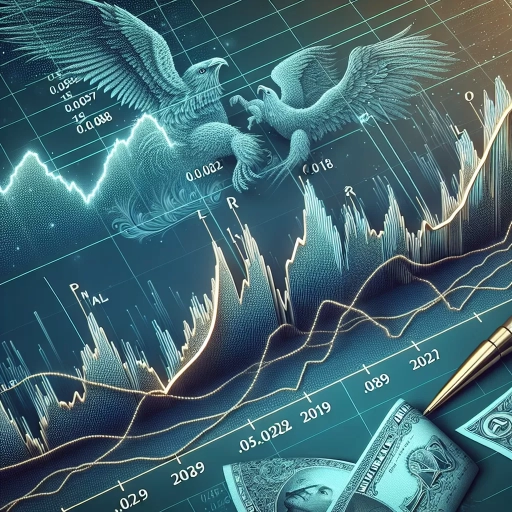