When Is Asr
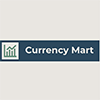
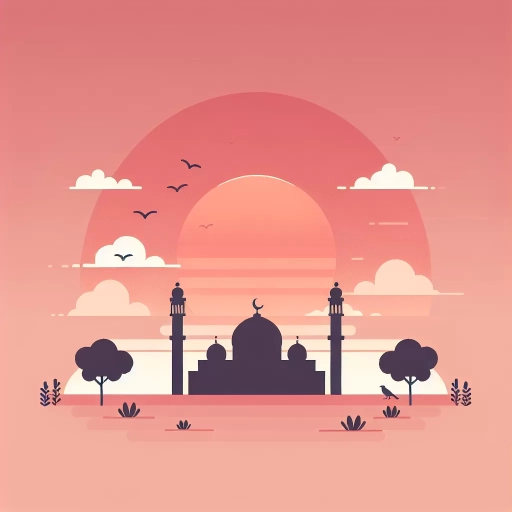
In the rapidly evolving landscape of artificial intelligence, Automatic Speech Recognition (ASR) has emerged as a transformative technology, revolutionizing how we interact with devices and access information. ASR enables machines to interpret and transcribe spoken language into text, opening up a myriad of possibilities across various sectors. This article delves into the intricacies of ASR, exploring its underlying technology, diverse applications, and the challenges it faces. We will begin by understanding the core principles of ASR technology, which forms the foundation of this innovative field. From there, we will examine the wide range of applications and use cases that leverage ASR, from virtual assistants and customer service systems to medical transcription and language learning tools. Finally, we will discuss the challenges and limitations that ASR still encounters, such as dealing with accents, background noise, and complex linguistic structures. By grasping these aspects, we can better appreciate the potential and current state of ASR. Let's start by diving into the fundamental concepts that make ASR possible: Understanding ASR Technology.
Understanding ASR Technology
In the rapidly evolving landscape of artificial intelligence, Automatic Speech Recognition (ASR) technology has emerged as a pivotal innovation, transforming the way we interact with machines. ASR, which enables computers to interpret and transcribe spoken language, has a rich history that spans several decades. From its early beginnings in the 1950s to the sophisticated systems of today, the **Historical Development of ASR** has been marked by significant milestones and technological breakthroughs. At the heart of modern ASR systems are **Key Components** such as acoustic models, language models, and deep learning algorithms, which collectively enhance the accuracy and efficiency of speech recognition. As we delve into the **Current State and Future Trends** of ASR, it becomes clear that this technology is not only improving but also expanding its applications across various industries. Understanding ASR Technology is crucial for appreciating its potential to revolutionize communication, enhance user experience, and drive innovation in fields like healthcare, education, and customer service. In this article, we will explore these facets in depth to provide a comprehensive understanding of ASR technology.
Historical Development of ASR
The historical development of Automatic Speech Recognition (ASR) technology is a narrative of continuous innovation and improvement, spanning several decades. The journey began in the 1950s with the first attempts at speech recognition, where simple systems could recognize a limited number of words and phrases. One of the earliest milestones was the development of the "Audrey" system by Bell Labs in 1952, which could recognize digits spoken by a single speaker. This pioneering work laid the groundwork for future advancements. In the 1960s and 1970s, ASR technology started to incorporate more sophisticated algorithms and statistical models. The introduction of dynamic time warping (DTW) in the 1960s allowed for better alignment between spoken words and reference patterns, significantly improving recognition accuracy. The 1970s saw the emergence of Hidden Markov Models (HMMs), which became a cornerstone in ASR due to their ability to model the temporal structure of speech. The 1980s marked a significant leap forward with the advent of neural networks. Although early neural network models faced challenges such as limited computational power and data availability, they paved the way for future deep learning approaches. The 1990s witnessed substantial improvements with the integration of Gaussian Mixture Models (GMMs) into HMM frameworks, enhancing robustness against various speaking styles and environmental conditions. The turn of the century brought about a revolution in ASR with the rise of deep learning techniques. Convolutional Neural Networks (CNNs) and Recurrent Neural Networks (RNNs), particularly Long Short-Term Memory (LSTM) networks, began to dominate the field. These models could learn complex patterns in speech data more effectively than their predecessors. The introduction of end-to-end models like Connectionist Temporal Classification (CTC) and sequence-to-sequence architectures further streamlined the recognition process. In recent years, advancements in computing power and large-scale datasets have propelled ASR technology to unprecedented levels of accuracy. The use of transfer learning from pre-trained models like BERT and Wav2Vec has significantly improved performance on diverse speech tasks. Additionally, the integration of attention mechanisms and transformer architectures has enhanced the ability to handle long-term dependencies in speech signals. Today, ASR technology is ubiquitous, powering applications such as virtual assistants, voice-controlled devices, and real-time transcription services. The historical development of ASR is a testament to human ingenuity and the relentless pursuit of technological advancement, transforming what was once a futuristic concept into an integral part of our daily lives. Understanding this evolution is crucial for appreciating the current state and future potential of ASR technology.