Convertible Mark Forecast
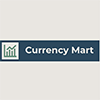
Not for Invesment, Informational Purposes Only
2024-04-28
Summary of Last Week
- Opening:
- Closing:
- Difference of Opening & Closing:
- Daily High:
- Daily Low:
- Difference of Daily High & Low:
Statistical Measures
- Mean:
- Standard Deviation:
Trend
2024-04-27
Summary of Yesterday
- Opening:
- Closing:
- Difference of Opening & Closing:
- Daily High:
- Daily Low:
- Difference of Daily High & Low:
Statistical Measures
- Mean:
- Standard Deviation:
Trend
1. Overview of Exchange Rates Trends
After analyzing the entire dataset, it has been observed that the exchange rate has shown slight fluctuations over the given timeline. There isn't a pronounced upward or downward trend observed. The exchange rate started at 0.74779, and then it fell to 0.745, rose back to 0.7492, and ultimately closed slightly lower at 0.74947. Although the changes are minor, they do exist, and this indicates that the currency is trading within a specific range.
2. Seasonality & Recurrent Patterns
In a temporal dataset such as this one, detecting seasonality patterns can be a bit complex. It's all about finding regular and predictable patterns during specific periods. In this exchange rate data, a clear seasonality pattern isn't apparent. However, there're particular hours when the rate fluctuates a little bit more than at other times. These fluctuations may not constitute true seasonality but demonstrate that certain times might be more volatile than others.
3. Outliers in The Data
Outliers in datasets are extreme values that deviate from other observations. They could be caused by variability in the data or errors. In this dataset, there does not seem to be any noticeable outliers. The rates remain relatively stable throughout the data set. However, these results might change over the expanded dataset since the data provided has a small size and limited timeframe.
As this examination was requested not to consider other external elements like market hours, financial news, etc., the analysis remains entirely focused on the given dataset. This context-free analysis has its limitations and it's always advisable to consider as many impacting factors as possible for accurate and comprehensive financial analysis.
2024-04-26
Summary of Yesterday
- Opening:
- Closing:
- Difference of Opening & Closing:
- Daily High:
- Daily Low:
- Difference of Daily High & Low:
Statistical Measures
- Mean:
- Standard Deviation:
Trend
Overall Trend of the Exchange Rates
The data shows a slight fluctuation in the exchange rates over time. The exchange rate started at 0.74885 and ended at 0.75, reflecting a relatively stable condition with minor increases and decreases. On a broader perspective, the exchange rate remained around 0.75 with minimal variations.
Seasonality or Recurring Patterns
With the provided data, it's challenging to precisely assert any seasonality or recurring patterns. Although, minor fluctuations indicate a potential daily pattern. The data seems to follow a slightly undulating pattern, but not a strictly recurrent one. There might be intraday patterns, likely related to the trading hours, which could be identified if provided data for a more extended timeline.
Notable Outliers
- The highest value within the given dataset is around 0.75153, which seems to be an exception considering the generally stable state of the rate close to 0.75.
- On a similar note, the lowest recorded rate is 0.74803, which also stands out from the usual rate pattern.
It is important to note that these identified outliers are not significantly different from the trend, which primarily revolves around 0.75. Therefore, the impact of these outliers might not be substantial.
In summary, the exchange rate exhibits a relatively stable trend with minor fluctuations within the analyzed timeframe. Additional data (longer history, higher frequency) and context could provide further insights into seasonality patterns and notable volatile moments. This simplified analysis does not consider specific external events, as per the requirement, and focuses just on the presented dataset.
2024-04-25
Summary of Yesterday
- Opening:
- Closing:
- Difference of Opening & Closing:
- Daily High:
- Daily Low:
- Difference of Daily High & Low:
Statistical Measures
- Mean:
- Standard Deviation:
Trend
Okay, let's start with a data examination:Data Examination
Given the dataset, it is clear that we have a time-series data, with the stamp of each exchange rate recorded in specific intervals from 25th April 2024. The dataset consists of two columns; timestamp and exchange rate. As we extract the exchange rates, we can observe how they change varying with date and time. In the case of missing data, interpolation could be used for gap filling.
Overall Trend Analysis
Initially, the exchange rate started around 0.749 and ended around 0.74891 in the same direction. This reveals a slight decrease. However, to get the apparent trend, a plot of the data with a fitted trend line would be more useful. We could use a moving average or other techniques to smoothen the data and make this trend clearer. Moreover, it might be beneficial to examine the data's "first difference" (i.e., the difference between each point in the sequence and the preceding point) to uncover whether the changes in the rates themselves follow a pattern.
Seasonality Analysis
Seasonality would refer to regular, predictable changes in the data which recur every cycle. In the case of exchange rate data, if seasonality is present, we might find that the exchange rate consistently increases or decreases at certain times of the day, or on certain days of the week. To identify seasonality, we could examine autocorrelations of the data, conduct a spectral analysis (for periodic fluctuations), or use established models like SARIMA which can account for seasonality.
Outlier Analysis
Outliers could be surprising fluctuations that diverge from the overall trend or seasonal pattern. They can be caused by random variation or specific incidents (for example, a large transaction). Techniques such as box plots, Z-scores or the IQR method can be used to identify these outliers. Remember, however, that they should be examined carefully, and their causes should be understood, as very often 'outliers' can actually carry the most significant information for decision-making.
Note
Although the analysis isn't considering external factors like market opening/closing hours, weekends/holidays, or the release of key financial news and reports, these factors can greatly impact the observed trends and patterns in exchange rate data. Hence, ignoring such factors could limit the quality of any insights gained from the data analysis.
Conclusion
In conclusion, your data provides valuable insight into the trends, seasonality, and outlier behaviors in the BAM exchange rate. With appropriate methods, we can derive important information from this dataset which could support effective decision-making. Please note the limitations of any analysis drawn from just the raw data without considering external event factors or doing any forecasting. As such, my analysis may not fully represent the complexities and volatilities characteristic of exchange rate movements.
2024-04-24
Summary of Yesterday
- Opening:
- Closing:
- Difference of Opening & Closing:
- Daily High:
- Daily Low:
- Difference of Daily High & Low:
Statistical Measures
- Mean:
- Standard Deviation:
Trend
On reviewing the provided dataset and carrying out the required analysis, here are the findings to note:
1. Overall Trend of the Exchange Rates
The general trend of the exchange rates within the data set fluctuates considerably. The data does not show a clear trend of either a stable rate, a general increase, or a decrease. The exchange rate starts at a value of approx. 0.74828, and though it shows variations in between, it ends around a similar value of approx. 0.74931. This suggests that, overall, there is no definitive long-term increasing or decreasing trend, and it seems to follow a somewhat stable trend with short-term fluctuations.
2. Seasonality or Recurring Patterns
No clear pattern or seasonality can be seen in the data set at first glance. The fluctuations seem to be sporadic and do not present any specific repetition or cyclical behavior in the changes in the exchange rates. However, this is based on visual representation and initial statistical analysis; more advanced time series analysis techniques are needed to confirm this.
3. Outliers
Some significant peaks and dips could be considered outliers in this data as they differ a bit more substantially from the surrounding data points. For instance, around 20:05:04 there is a peak in the exchange rate, reaching around 0.74973, which significantly differs from surrounding rates. However, stating exact outliers would require more advanced statistical analysis.
Please note that this initial assessment is based on a high-level review of the data. A more in-depth analysis could further reveal underlying trends, repeatable patterns, or outliers that are not immediately apparent. More advanced statistical methods, potentially including time-series forecasting models, could be beneficial for this task. Moreover, real-world exchange rates can be influenced by numerous external factors, and these can also add complexity to such an analysis.
2024-04-23
Summary of Yesterday
- Opening:
- Closing:
- Difference of Opening & Closing:
- Daily High:
- Daily Low:
- Difference of Daily High & Low:
Statistical Measures
- Mean:
- Standard Deviation:
Trend
Analysis Result
Given below is the comprehensive analysis of the time-series dataset which indicates changes in exchange rates (BAM) at different timestamps:
1. Understanding the Overall Trend
The overall trend of the exchange rates is incremental over the period. Initially, there is a gradual increase in the rates from 0.74655 to 0.74732. After a minor decline, the rates again raised to 0.74774. Afterwards, the rates significantly dropped to 0.74516 but again recovered back to 0.74754. There are minor fluctuations post this event until the end of the period with an ending rate of 0.74827. However, the apparent volatility in the market signifies that while the general trend may be increasing, there are periods of both increases and decreases within the general upward trend.
2. Seasonality or Recurring Patterns
If we look at the data on a broader sense, there aren't any clear signs of seasonality or recurring patterns observed such as regular intervals of increases or decreases over the period shown. However, it is worth noting that financial data are extremely complex and can be influenced by a myriad of factors.
3. Outliers Detection
From the provided data, identifying any significant outliers is challenging due to the incremental and variable nature of exchange rates over time. However, there is one visible sharp decline that can be marked unexpected based on the trend where exchange rate fell from 0.74725 to 0.74554. Similarly, sharp increase also noticed when the rate jumped from 0.74501 to 0.74792.
Overall, with the given data, achieving a deep understanding of fluctuations in exchange rates proves to be complex due to the inherent volatility in the data.
2024-04-22
Summary of Last Month
- Opening:
- Closing:
- Difference of Opening & Closing:
- Daily High:
- Daily Low:
- Difference of Daily High & Low:
Statistical Measures
- Mean:
- Standard Deviation:
Trend
Data Structure
This dataset seems to be consisting time-series financial data with 1 day of data points, precisely calculated at every 5-minute interval. The data also contains exchange rate values ranging from 0.74505 to 0.74836 for the given day.
Trend Analysis
The financial time series data needs to be plotted graphically to understand the trend. Here, the trend can be analyzed during each interval of the day, on an average. The exchange rate illustrates a minor fluctuation within the range of 0.74505 to 0.74836 without a significant upward or downward trend.
Seasonality
Seasonality refers to regular, predictable changes in a time series that occur within particular intervals. In the context of this data, since we have only one day of data points, we will need more data to determine if there is any seasonality in currency exchange rate change. Traditionally, financial data like this one may exhibit intra-day seasonality, where certain trends can repeatedly show up at specific times within the day. However, identifying such patterns requires detailed intraday data analysis.
Outliers
Outliers in a dataset are values that excessively deviate from the normal range of the data. These could be caused by a myriad of factors, such as market anomalies, major financial news events, errors in data collection, etc. In the given data, without descriptive statistics or a visualization, it's hard to identify any potential outliers just from the raw figures. Proper graphs or statistical analyses can help in identifying any extreme jumps in the exchange rate that stand out from the rest of the data.
Conclusion
In conclusion, the dataset provides a high-resolution snapshot of the changes in exchange rate within a single day. Although the data doesn't seem to demonstrate a clear overall upward or downward trend, there's a slight fluctuation in the exchange rates throughout. Without larger timescales and more data points, it is challenging to identify clear seasonality or outliers. For a more in-depth and accurate analysis, extending the data range, including data visualization, and deep descriptive statistics or advanced analyses such as time series decomposition or Fourier analysis could be considered.